Executive Summary
Machine learning can make the additive manufacturing (AM) process for multi-material metal printing cheaper and faster and support the production of complex, high-performance, cost-effective parts for energy, automotive, and aerospace industries. Exponential Technologies together with Aerosint developed parameter sets for multi-material laser powder bed fusion (LPBF) for the Stainless Steel 316l and Inconel 625 combination. Within 2 print jobs and 46 samples printed and tested xT SAAM was able to improve upon the initial parameter set by 2x resulting in denser parts with a much higher printing speed. The project team was able to achieve the final parameter set with no need for statistical knowledge. This shows how xT SAAM machine learning can dramatically speed up AM process parameter optimization, save time and money on materials, increase time-to-market and reduce R&D costs.
Multi-Material LPBF
To achieve the highest flexibility and high-performance parts multi-material 3d printing is necessary. This is why Aerosint has developed a Selective Powder Deposition (SPD) recoater that enables multi-metal LPBF 3D printing. Aerosint chose to use xT developed AI platform xT SAAM for the development of Stainless Steel 316l and Inconel 625 material combinations as traditional methods such as DoE have shown to be expensive and time-consuming. Combining these materials in a single part would allow the manufacturing of better cost-performing parts with high complexity and corrosion and oxidation resistance. These parts would be able to perform well in extreme environments under high mechanical loads and at high temperatures. This would be of great use in the energy, automotive, and aerospace industries.
Combining Stainless Steel 316l and Inconel 625
The aim of the project was to find a single process parameter set for the combination of Stainless Steel 316l and Inconel 625 metals to maximize part density and build rate. This was possible as both materials can have an overlap of processing window.
Aerosint used Aconity 3d printers in combination with their recoaters and developed settings for them using xT SAAM platform by printing cubes and measuring their density using 2D optical images of the cross-section. Parameters such as laser power (150-400W), scanning speed (400-2000 mm/s) and hatch distance (70-140μm) were varied while layer thickness was kept constant.
For the development of parameter settings, Aerosint used xT SAAMs active learning module together with automated ensemble modeling. Combining these 2 methods allows xT SAAM’s users to drastically reduce the number of experiments needed in R&D and process development and get the most out of their data.
The active learning method is based on genetic algorithms that suggest new parameter settings based on existing data and objectives. In this approach, our proprietary genetic algorithms make suggestions that improve with each experimental cycle. The automated ensemble modeling method is based on finding the best combination of machine learning models for a given data set to maximize predictive accuracy.
Solution
The optimization process began with adding existing data of the process that Aerosint had on hand based on available literature. The initial data consisted of 8 different printing recipes. The best one achieved a relative density of 99.7% and a build rate of 2.88 mm3/s
Using available data xT SAAM software made 40 different printing recipes for the second iteration, both recipes with small changes to the existing parameters and completely new combinations. The second iteration was able to both improve the density of the end parts to over 99.9% and drastically improve the build rate by 36% to 3.94 mm3/s
Now based on available 48 data points (8 from initial data and 40 based on series 2) both optimization and modeling could be used to get suggestions for new parameter combinations. For the second iteration, feedback was given for 6 new parameter suggestions with incredible results, improving the build rate even more by 54% reaching 6.08 mm3/s while keeping density above 99.9%.
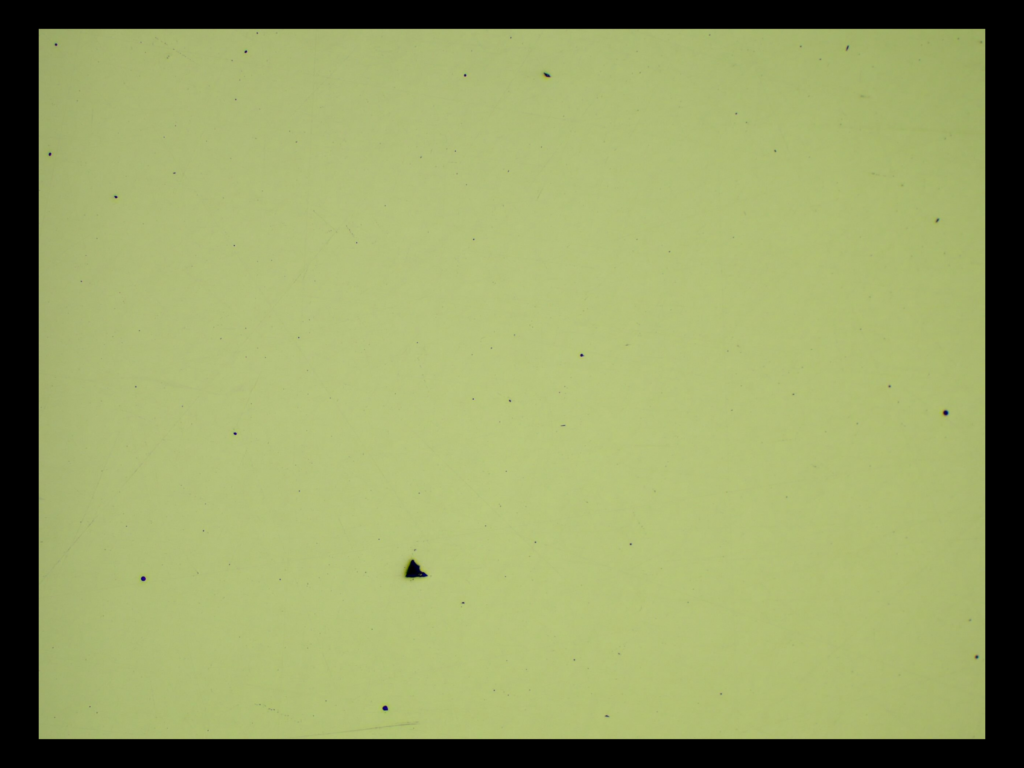
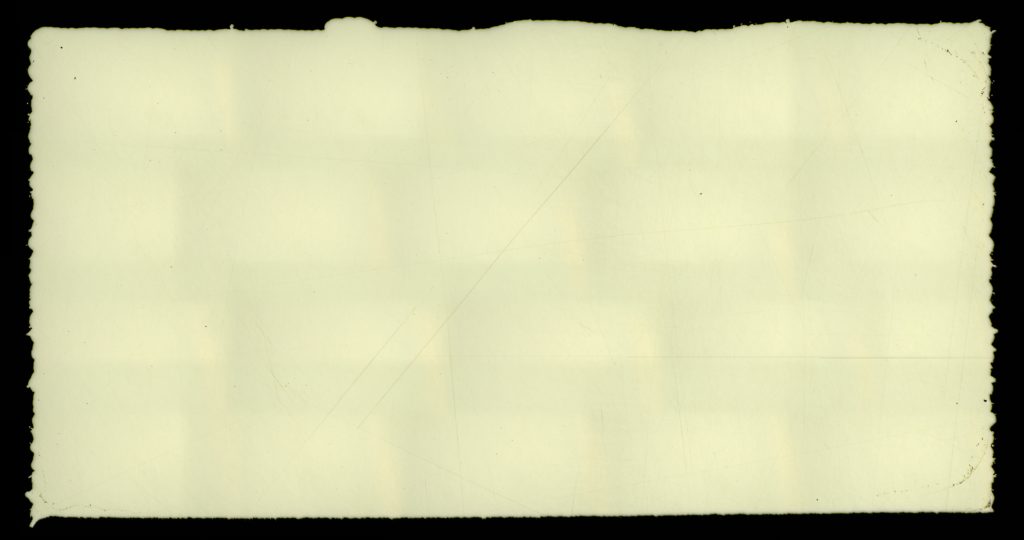
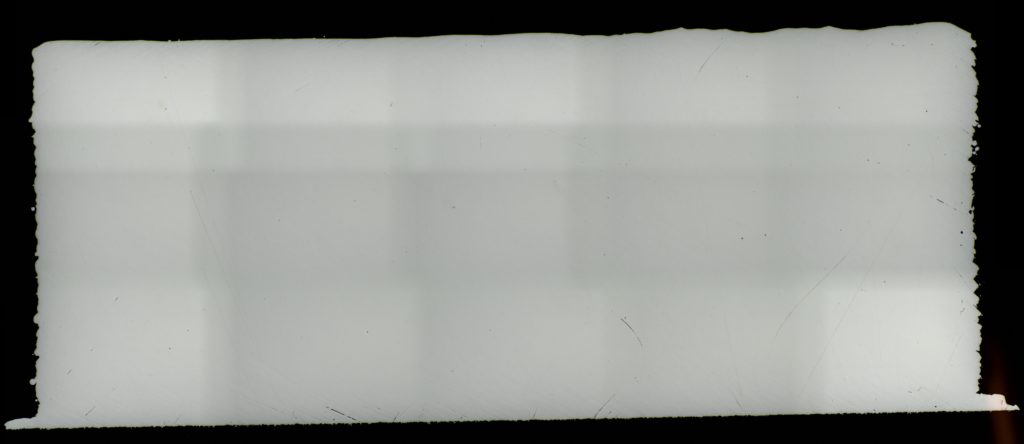
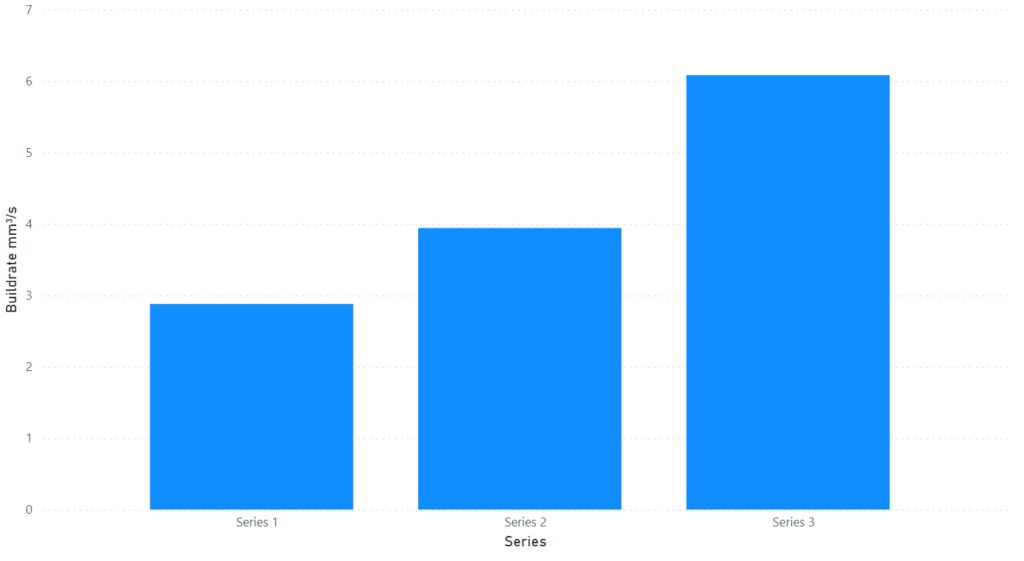
Modeling helps with understanding the problem space and possible directions where to find the best results. It can be used to explain the stability of process parameters for usage in production. With a cross-validated R2 of 0.4, there is much more data that would be needed to be able to fully explain the process, but certain tendencies can be observed.
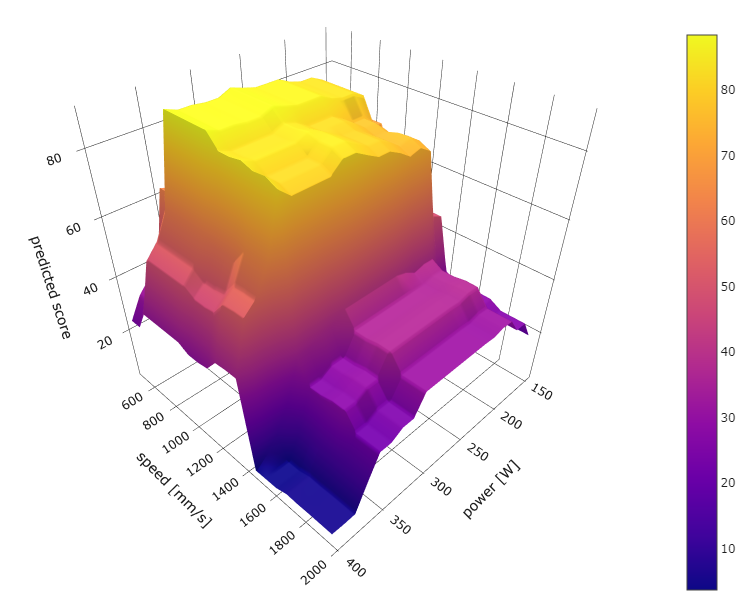
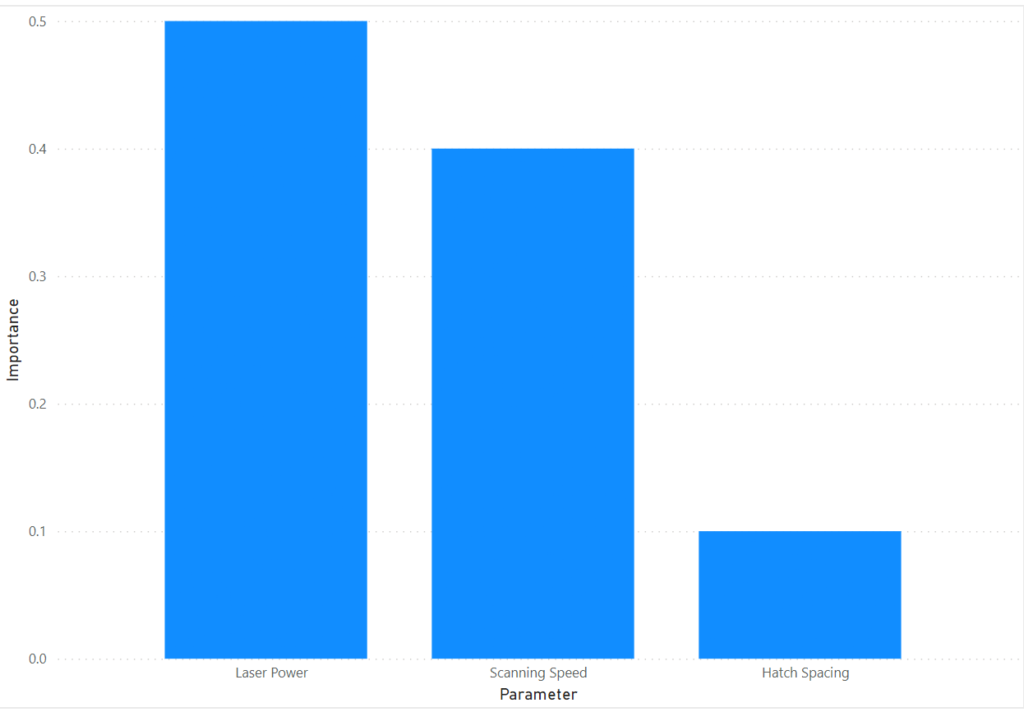
“The xT SAAM software is a powerful tool for developing processing parameters for not only single-material but also multi-material additive manufacturing. The development cycle was significantly reduced to achieve high density and high productivity. The results from this collaboration will be used in our future manufacturing of samples for scientific research as well as industrial demonstrators. To apply these fruitful results to the right products and industries, we are looking forward to further advance the collaboration with Exponential Technologies.“
Dr. Xiaoshuang Li, Head of LPBF Process & Applications
Amazing Results
Within 2 print jobs and 46 samples printed and tested xT SAAM was able to improve upon the initial parameter set by 2x resulting in denser parts with a much higher printing speed. The initial printing parameters that resulted in a speed of 2.88 mm3/s were far exceeded with 2nd iteration samples being printed at over 6.08 mm3/s while keeping densities over 99.9%. Most importantly, all developed parameter sets were vastly different from each other. This variety of parameter sets allows switching between parameter sets based on priority or higher production stability. The project showed that our xT SAAM platform helps to reduce R&D efforts and increases part quality and production speed compared to currently used methods such as Design of Experiments (DoE)
It is a great achievement to identify the overlap of the processing window of two dissimilar materials assisted by machine learning. This allows the utilization of continuous scanning across the material interface instead of sequential scanning. In other words, even though there are two different powders on each layer, the slicing and hatching can be performed as it is routinely done for a single material. It significantly reduces the work for build job preparation and improves the process robustness and part quality.
Why does it matter?
xT SAAM, an easy-to-use machine learning software for R&D helps optimize and develop products and processes without the need for advanced statistical or programming knowledge. Users using their domain expertise together with xT SAAM can:
- Save time and money on materials by reducing the number of experiments
- Reduce overall R&D costs
- Decrease time-to-market
Selective Powder Deposition system allows additive manufacturing of multi-material products in a single step. In addition to the inherent geometrical complexity from additive manufacturing, functionality freedom is brought to product, which further drives the production cost down while improving part performance. The vision is to manufacture innovative products with region specific properties by joining different materials. Using the xT SAAM can significantly facilitate the process development for multi-material additive manufacturing and productivity improvement.
Exponential Technologies
Exponential Technologies is an experienced player in the application of artificial intelligence (AI) and machine learning (ML) in industrial markets. In particular, xT has a track record of delivering world-class software products in the field of industrial R&D. Combining their experience with AI/ML technologies and their knowledge of the AM market, xT is ready to gradually turn the promise of “first time right” into reality.
Aerosint SA
Based in Belgium, Aerosint SA is now part of Desktop Metal from USA. The patented innovation developed by Aerosint is an alternative powder recoating system that selectively deposits up to three powders to form a single layer. It enables full 3-dimensional control over material placement in any powder bed additive manufacturing process such as binder jetting, SLS, LPBF and so on. Therefore, this technology could be integrated into manufacturing processes where a specific powder pattern is needed. In the additive manufacturing process, Aerosint has partnered with the German 3D printer manufacturer “Aconity 3D GmbH”, offering multi-material LPBF printers to customers globally. Aerosint’s customers are from a wide range of industries such as aerospace, industrial tooling, automotive, watch industry, injection molding etc.